Predictive Models for TBM Penetration
- SAALG GEOMECHANICS
- 5 days ago
- 2 min read
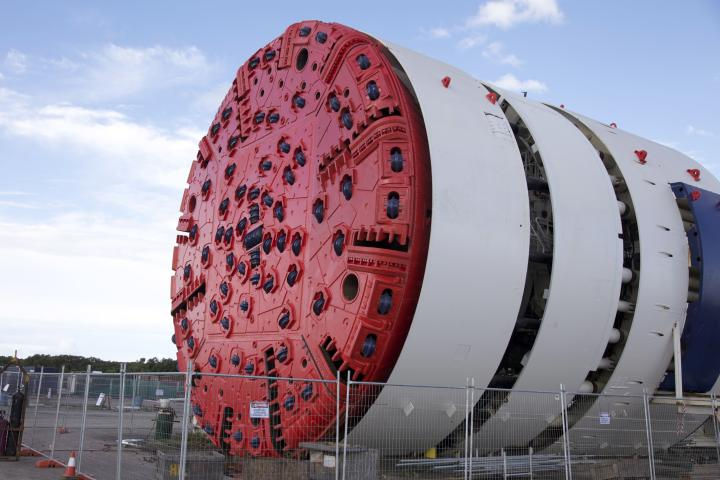
Mechanized tunneling using Tunnel Boring Machines (TBMs) is one of the most advanced and efficient methodologies in modern geotechnical engineering. The ability to predict the TBM penetration rate (PR) is fundamental for planning, risk management, and production optimization in underground works. In this context, predictive models powered by real-time data and machine learning algorithms have transformed how excavation processes are analyzed and controlled.
Technical background of penetration rate
PR is defined as the amount of TBM advance per unit of time, typically expressed in meters per hour. It is influenced by several factors, including:
Ground properties: unconfined compressive strength (UCS), abrasivity, cohesion, water content, and geological structure.
Operational parameters: thrust, torque, cutterhead RPM, chamber pressure, advance speed.
External conditions: stratigraphic variability, structural interferences, and obstacles.
The key challenge lies in the complex and nonlinear interaction among these factors, which vary continuously along the tunnel alignment.
Limitations of traditional models
Classic approaches—such as Graham’s method, NTNU’s model, or empirical industry-based correlations—provide a starting point but are static and non-adaptive. Their accuracy depends heavily on the quality of pre-construction data, which can be limited, especially in urban environments or geologically heterogeneous conditions.
Data-driven predictive models
The digital revolution enables real-time integration of TBM sensor data. Variables like torque, thrust, penetration rate, cutterhead rotations, and shield pressure are continuously logged. Combined with geotechnical characterization data (boreholes, in-situ and lab tests), these datasets feed machine learning models that detect performance patterns and predict PR based on ground conditions.
Commonly used techniques include multivariate regressions, artificial neural networks, decision trees, and hybrid models that blend physical rules with statistical learning. Unlike deterministic approaches, these models automatically adapt to real behavior and support dynamic updates.
Operational advantages
Accurate performance predictions along different geological sections
Better decision-making for advance parameters and control strategy
Early anomaly detection and proactive risk mitigation
Improved maintenance and logistics planning
Integration with numerical models for ground parameter recalibration
DAARWIN: Intelligence for tunnel excavation
DAARWIN integrates TBM operational data with machine learning–based predictive models. Through a cloud-based platform, technical teams can visualize PR trends, compare predictions with actual results, and adjust excavation strategies in real time.
A key feature is automatic recalibration of penetration models ring-by-ring, supported by integrated geotechnical backanalysis. DAARWIN transforms tunneling into a dynamic, adaptive process aligned with the principles of the Observational Method.
Additionally, DAARWIN supports retrospective analytics to identify anomalous behavior zones, trace root causes, and optimize strategies for future designs and constructions.
The application of predictive models to TBM penetration represents a shift toward data-driven decision-making. Tools like DAARWIN demonstrate that digitalization not only improves operational efficiency but also enhances safety, control, and adaptability to real ground conditions. This new tunneling paradigm empowers engineers to anticipate, optimize, and build with greater confidence.
👉 Want to see how this works in action? Explore more about TBM Data Management with DAARWIN.