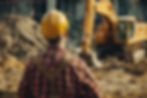
Geotechnical engineering stands as a cornerstone in the realm of civil engineering, playing a pivotal role in infrastructure development, construction projects, and environmental management. Over recent years, the integration of machine learning techniques has sparked significant interest and advancement within the field. This article delves into the synergy between machine learning and geotechnical engineering, elucidating the transformative potential of this amalgamation.
Understanding Machine Learning: Machine learning, a subfield of artificial intelligence, empowers systems to learn patterns and make predictions from data without explicit programming. Through algorithms and statistical models, machine learning facilitates the extraction of actionable insights from complex datasets. Key techniques include supervised learning for classification and regression tasks, unsupervised learning for clustering and dimensionality reduction, and reinforcement learning for decision-making in dynamic environments.
Integration of Machine Learning in Geotechnical Engineering: Machine learning techniques have revolutionized various facets of geotechnical engineering, enhancing traditional methodologies and enabling new approaches. For instance, in site characterization and soil classification, machine learning algorithms can analyze geospatial data, geological surveys, and laboratory test results to categorize soil types and determine their engineering properties. Moreover, AI-driven machine learning models aid in predicting soil behavior under different loading conditions, guiding foundation design and construction planning.
Real-world applications highlight the effectiveness of machine learning in enhancing geotechnical engineering processes. For example, in slope stability analysis, machine learning algorithms can analyze historical slope failure data along with geological and environmental factors to develop predictive models. These models enable engineers to assess the risk of slope instability more accurately and implement targeted mitigation measures.
Furthermore, in groundwater flow modeling and contaminant transport prediction, machine learning techniques can analyze complex hydrogeological datasets to forecast the movement of groundwater and pollutants in the subsurface. By incorporating machine learning into groundwater management strategies, engineers can optimize remediation efforts, minimize environmental impacts, and safeguard public health.
Additionally, in the realm of infrastructure monitoring and maintenance, machine learning algorithms can analyze sensor data from geotechnical instruments such as inclinometers, piezometers, and settlement gauges to detect anomalies and predict potential failures. This proactive approach to asset management enables infrastructure owners to prioritize maintenance activities, extend the lifespan of structures, and ensure the safety and reliability of critical infrastructure systems.
Benefits and Challenges: The integration of machine learning into geotechnical engineering offers a myriad of benefits, ranging from better risk management and decision-making to cost savings through optimized designs and construction processes.
One significant advantage is the enhancement of risk management and decision-making capabilities. Machine learning algorithms can analyze vast amounts of geotechnical data, including soil properties, geological characteristics, and historical performance data, to identify patterns and predict potential risks. By leveraging these insights, engineers can make more informed decisions regarding site selection, foundation design, and construction techniques, ultimately reducing the likelihood of costly delays, failures, and safety hazards.
Moreover, machine learning facilitates cost savings through optimized designs and construction processes. By iteratively analyzing design parameters, materials properties, and environmental conditions, machine learning models can identify optimal solutions that minimize construction costs while ensuring structural integrity and performance. These optimized designs not only reduce project expenses but also enhance efficiency and sustainability, aligning with the principles of lean construction and resource conservation.
However, despite the numerous benefits, the implementation of machine learning in geotechnical engineering is not without its challenges. One major challenge is data availability. Geotechnical data is often sparse, heterogeneous, and subject to uncertainty, posing challenges for training machine learning models effectively. Addressing this challenge requires efforts to collect, standardize, and curate high-quality geotechnical datasets, as well as developing techniques for data augmentation and synthesis to supplement limited data sources.
In conclusion, the integration of machine learning into geotechnical engineering represents a significant step forward in enhancing the efficiency, accuracy, and sustainability of infrastructure development projects. As highlighted throughout this article, machine learning algorithms have demonstrated their effectiveness in various aspects of geotechnical engineering, from site characterization and soil classification to slope stability analysis and groundwater flow modeling.
One notable platform that exemplifies the convergence of technology and geotechnical engineering is Daarwin. Daarwin offers innovative solutions for digitizing borehole data, visualizing geotechnical information, and analyzing design options with different ground parameter scenarios. By leveraging machine learning algorithms, Daarwin enables engineers to make informed decisions based on reliable ground information, optimize designs, and monitor construction progress more effectively.
Furthermore, Daarwin's learning algorithms facilitate the analysis of multiple design options and ground parameter scenarios, allowing engineers to determine the optimal design solution and assess its performance against monitoring data. This iterative process empowers engineers to refine designs, mitigate risks, and also minimizes construction costs by eliminating unnecessary expenses and ensuring optimal resource utilization.
In essence, Daarwin exemplifies the transformative potential of machine learning in geotechnical engineering, enabling engineers to harness the power of data-driven insights and predictive analytics to address complex challenges and optimize project outcomes. As technology continues to evolve and innovation accelerates, the synergy between machine learning and geotechnical engineering holds promise for driving sustainable development and resilience in infrastructure projects around the world.